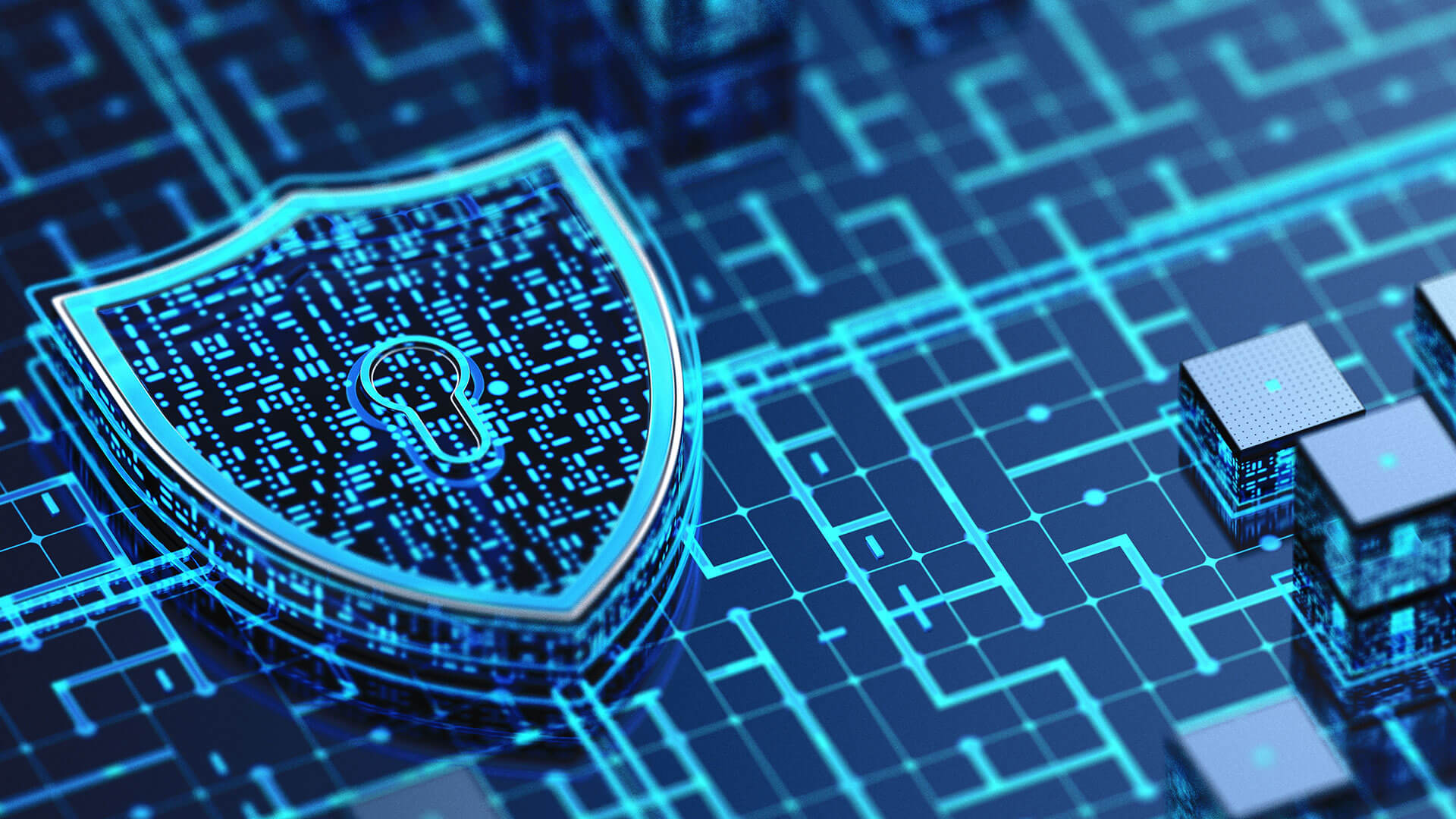
Safeguarding the responsible, safe, and human-centric development of artificial intelligence is paramount in unlocking its vast social and economic potential.
Considered as the next industrial revolution, AI is poised to change almost everything in our lives but understanding and mitigating the risks - many of which are not fully understood - is a deeply complex and difficult topic to address.
As governments around the world rush to establish frameworks for identifying, ranking, and managing these risks, Intel, a global leader in the advanced chip technology driving the AI revolution, emphasises the importance of considering the entire ecosystem.
One way to help ensure this technology is used to advance societal goals, is by embedding security and safety into the fabric of the software and hardware tools that are used to create new AI applications.
Putting security and ethical development at the centre of product development reduces risks associated with data protection, intellectual property theft and bias. This requires more than technical skills, says Intel, it requires a comprehensive approach to people, process, data collection and algorithmics.
Increasingly the risks of AI are being discussed more on a global stage by governments and industry representatives. As regulatory frameworks come into practice, it’s crucial we are thinking about what they could look like and apply those rules to tech development today. We are always asking ourselves, where are the risks and how can we as a multinational play our role,” said Walter Riviera, machine learning engineer at Intel.

In order to identify and mitigate risks as AI technologies continue to evolve, Intel has established advisory groups that comprise a diverse range of stakeholders, such as representatives from civil society, legal experts, academics, and government officials. These advisory groups provide guidance throughout all phases of product development.
“Everyone is moving fast in this area. We know we need to work together in a collaborative way to ensure the explosive growth of AI is geared towards bettering society and the community. It’s very much about international collaboration.”
As a key player in this AI ecosystem, we want to show people the good AI can bring to society and drive adoption across it,” Riviera said.
Approaching technology development in this way has also led to tangible product development outcomes in areas like confidential computing, designed to protect data in use through encryption, isolation, and attestation. This includes silicon-enabled security technologies like Intel® Trust Domain Extensions (Intel® TDX) and Intel® Software Guard Extensions (Intel® SGX).
Scenarios where these features can play a critical role include data security and IP protection, privacy and compliance, data sovereignty and control, and confidential AI. Hardware-enabled security boosts protection and enables the ecosystem to better defend against evolving and modern cybersecurity threats.
Federated learning: Providing benefits while protecting privacy
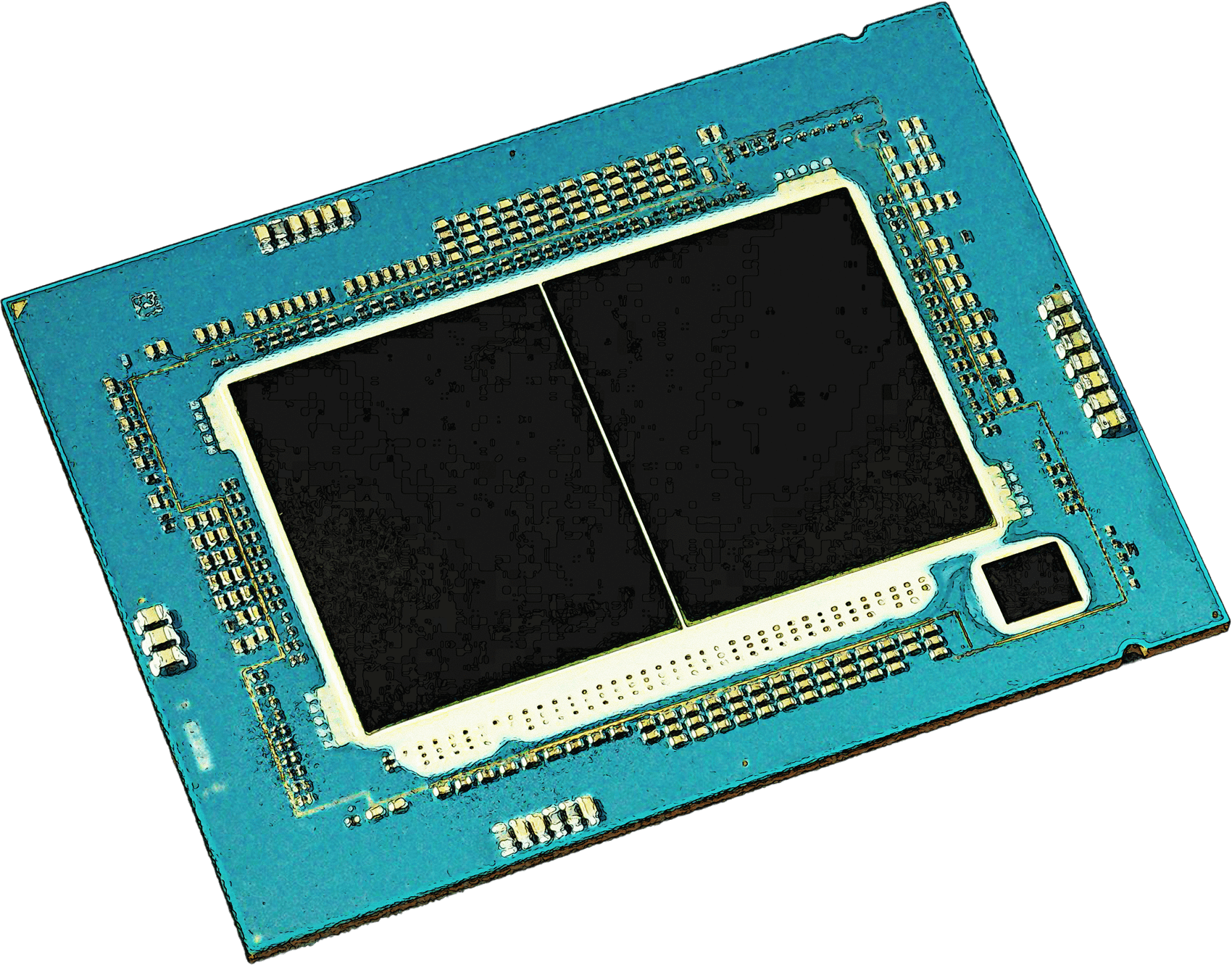
Whilst there is a lot of emphasis on Generative AI (large language models) such as ChatGPT, there are many other ways in which Machine Learning is being trained and deployed to help improve the outcomes in industries, such as healthcare.
In 2022, Intel Labs collaborated together with The Perelman School of Medicine, University of Pennsylvania to apply a machine learning technique known as federated learning to improve the speed and accuracy of brain tumour diagnosis.
The algorithm, trained on health data from around the world, was able to improve the identification of malignant brain tumours by 33 per cent. It was the largest medical federated learning study to date with a global dataset examined from 71 institutions across six continents.
But what is federated learning and how does this approach apply to Machine Learning?
Federated learning is an approach to machine learning that addresses the data access problem, for instance when data is protected by privacy or when it is stored in a remote location where downloading might not be possible.
This technique can leverage data from multiple, independent sources but it does not require the owners of the data, in this case hospitals, to export or share the data. This approach protects the privacy and ownership of patient data from across the 71 institutions.
Federated learning techniques offer society new solutions to complex issues. The machines treat the datasets as stand alone, so the legal ownership still stays with the contributor. This is different to traditional ways of managing data that merge datasets together and that’s a very important difference,” Riviera said.
“Adhering to data privacy laws whilst unlocking the power of AI with vast datasets is remarkable - and whilst we need to talk about safety and regulation, at the same time, we need industries that can benefit from AI to understand what applications can do in a safe way.”
This technique is already in place among financial institutions and will play a major role for smart environments and smart cities.”
Governing technology internally: Intel processors and data protection

Building roadmaps and having a clear set of standards in product development, not just for the protection of data but also for intellectual property, are a core part of Intel’s security and safety strategy.
Establishing advisory boards with a diverse range of stakeholders to develop these standards, whilst also learning from international standards, other AI ecosystems and anticipating regulatory requirements, is vital, says Intel.
We listen to issues around safety in AI and try to turn that into technical language when we develop our products. As well, we believe in diverse collaboration with external partners and legal consultancy for any AI project to ensure a holistic view of governance.”
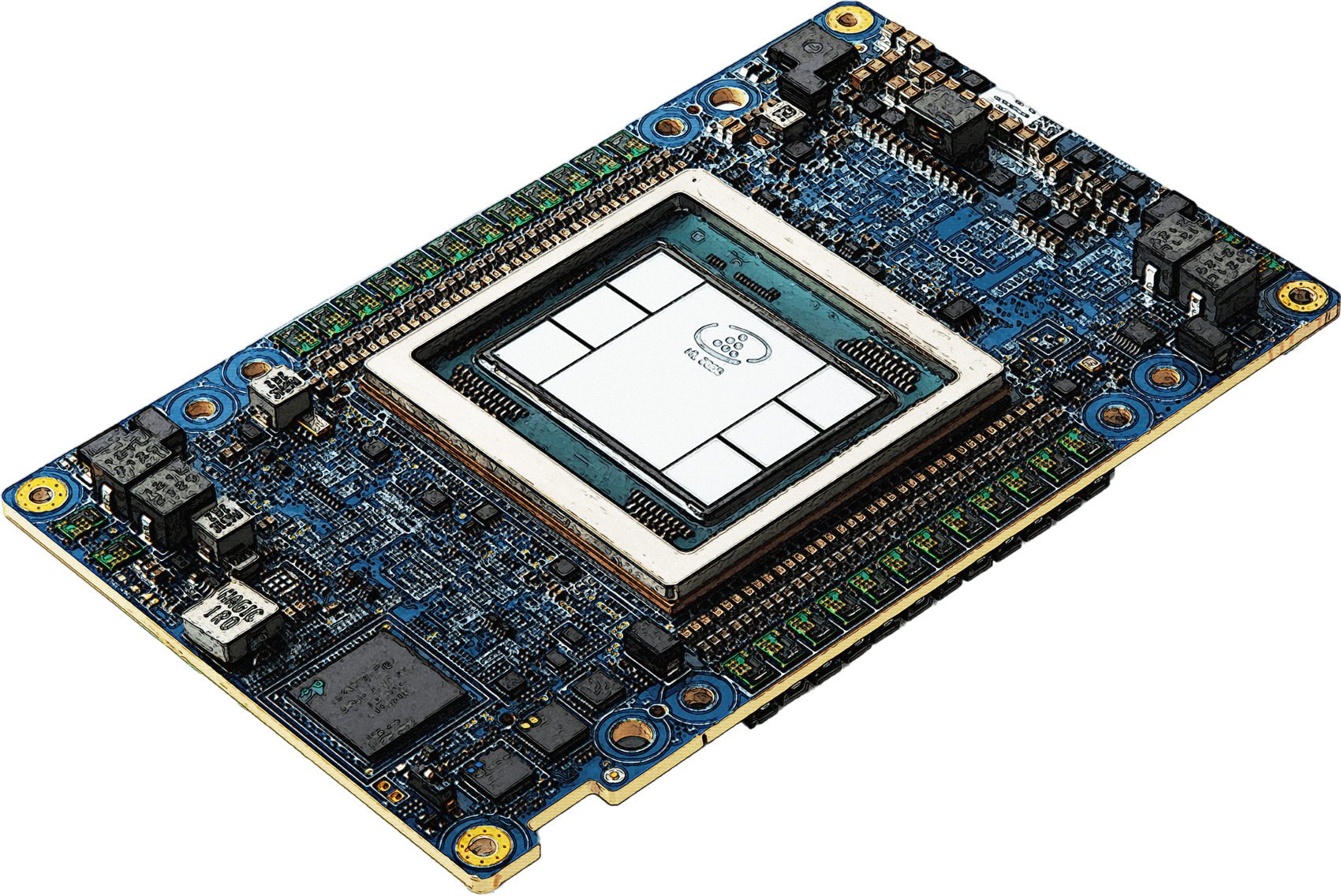
Intel’s cutting-edge hardware can help with the various steps of an AI pipeline. For instance, Intel Xeon Scalable processors include features like Intel® Advanced Matrix Extensions (Intel® AMX) to accelerate AI workloads for both training and inference. Intel® Data Center GPU Max Series for AI training, HPC and graphics workloads or the Intel® Data Center GPU Flex Series, ideal for media processing, cloud gaming or AI visual Inference and virtual desktop infrastructure.
The Deep Learning dedicated Intel Gaudi AI Accelerator, ideal for Generative AI workload as demonstrated by the 2x Performance Leap on GPT-3 with FP8 Software adoption for Stable Diffusion - a deep learning text-to-image generator - have enormous computing power and are being used by some of the biggest creators of AI.
As more high-profile companies deploy Intel processors for AI, verifying and protecting the data’s integrity, confidentiality and authenticity becomes as critical as the technical capabilities of the processors.
We think about how to protect the data being processed by AI and this includes threats to the most sensitive data - which have become increasingly advanced - creating risks for companies, government organisations, and cloud providers.”
“Ensuring hardware and software-based security solutions are built in at every stage for cloud and enterprise environments is critical because everyone in the value chain is responsible for equitable use of AI.”
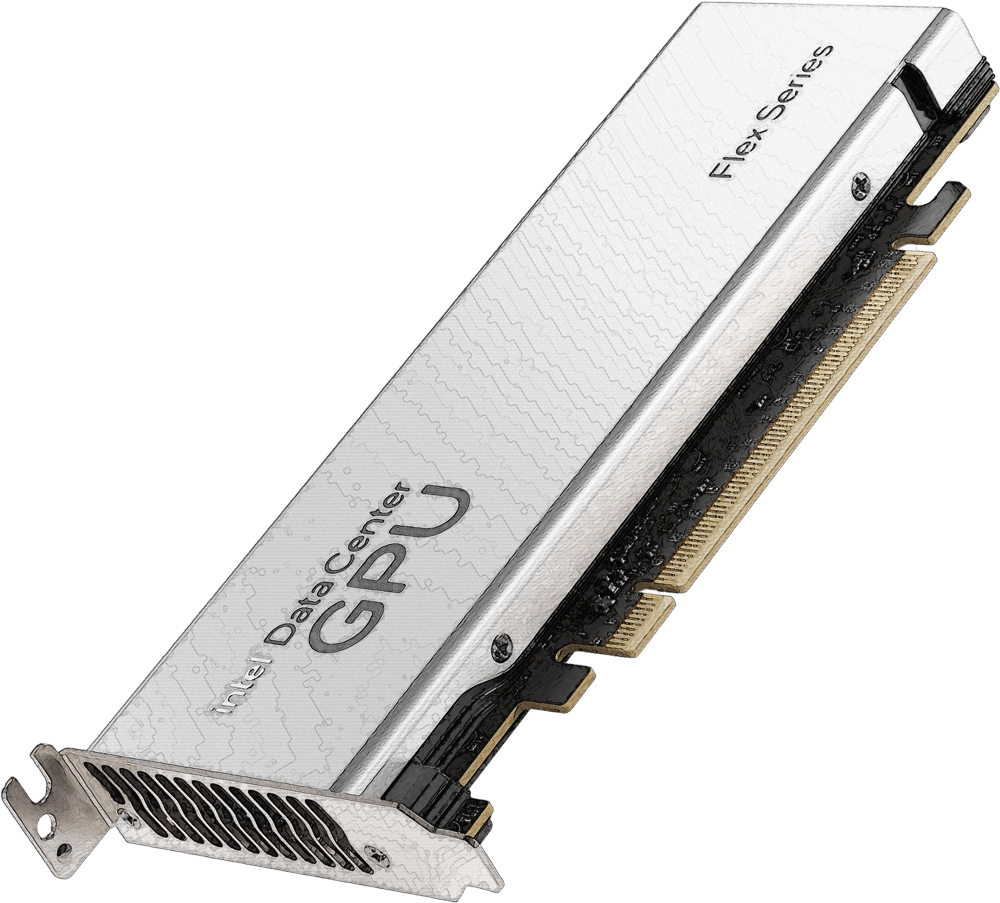
Global collaboration on building AI for good
As artificial intelligence continues to impact all industries around the world, the need to collaborate and agree on risk factors and ways to manage those risks will only grow.
However, the call to develop more use cases and applications that benefit all levels of society economically and socially is just as important. This is the case for leaders in AI technology such as the US, Europe and China but also across developing nations in Africa and Latin America.
“There are endless possibilities for AI. It’s about responsible innovation and building the right regulation at each step. Transparency is really important and I think the key players such as governments, think tanks, industry - that understand that.”
The more examples of AI for good that are out there and deployed in a safe and secure way, the more we will see industry - not just large companies, but SMEs and civil society, really make the most of this and create a future that we all want to live in.”